plotResidualsDistribution
[Monolix] Generate Distribution of the residuals
Plot the distribution of the residuals.
Usage
plotResidualsDistribution(
obsName = NULL,
residuals = c("indiv", "npde"),
plots = c("pdf", "cdf"),
settings = list(),
preferences = list(),
stratify = list()
)
Arguments
- obsName
(character) Name of the observation (in dataset header). By default the first observation is considered.
- residuals
(character) List of residuals to display: population residuals ("pop"), individual residuals ("indiv"), normalized prediction distribution error ("npde") (default c("indiv", "npde)).
- plots
Type of plots: probability density distribution ("pdf"), cumulative density distribution ("cdf") (default c("pdf", "cdf")).
- settings
List with the following settings
indivEstimate
(character) Calculation of individual estimates: conditional mean ("mean"), conditional mode with EBE's ("mode"), conditional distribution ("simulated") (default "simulated").useCensored
(logical) Choose to use BLQ data (TRUE) or to ignore it (FALSE) to compute the statistics (default TRUE). For continuous data only.censoring
(character) BLQ data can be simulated ('simulated'), or can be equal to the limit of quantification ('loq') (default 'simulated'). For continuous data only.legend
(logical) add (TRUE) / remove (FALSE) plot legend (default FALSE).grid
(logical) add (TRUE) / remove (FALSE) plot grid (default TRUE).ncol
(integer) number of columns when facet = TRUE (default 4).fontsize
(integer) Plot text font size.
- preferences
(optional) preferences for plot display, run getPlotPreferences("plotResidualsDistribution") to check available displays.
- stratify
List with the stratification arguments:
groups
- Definition of stratification groups. By default, stratification groups are already defined as one group for each category for categorical covariates, and two groups of equal number of individuals for continuous covariates. To redefine groups, for each covariate to redefine, specify a list with:name character covariate name (e.g "AGE"
)definition (vector(continuous) || list>(categorical)) For continuous covariates, vector of break values (e.g c(35, 65)
). For categorical covariates, groups of categories as a list of vectors(e.glist(c("study101"), c("study201","study202"))
)split
(vector) - Vector of covariates used to split (i.e facet) the plot (by default no split is applied). For instancec("FORM","AGE")
.filter
(list< list> >) - List of pairs containing a covariate name and the vector of indexes or categories (for categorical covariates) of the groups to keep (by default no filtering is applied). For instance,list("AGE",c(1,3))
to keep the individuals belonging to the first and third age group, according to the definition ingroups
. For instance,list("FORM","ref")
using the category name for categorical covariates.
Value
A ggplot object if one prediction type,
A TableGrob object if multiple plots (output of grid.arrange)
See also
Examples
initializeLixoftConnectors(software = "monolix")
project <- file.path(getDemoPath(), "1.creating_and_using_models",
"1.1.libraries_of_models", "theophylline_project.mlxtran")
loadProject(project)
runPopulationParameterEstimation()
runConditionalDistributionSampling()
plotResidualsDistribution()
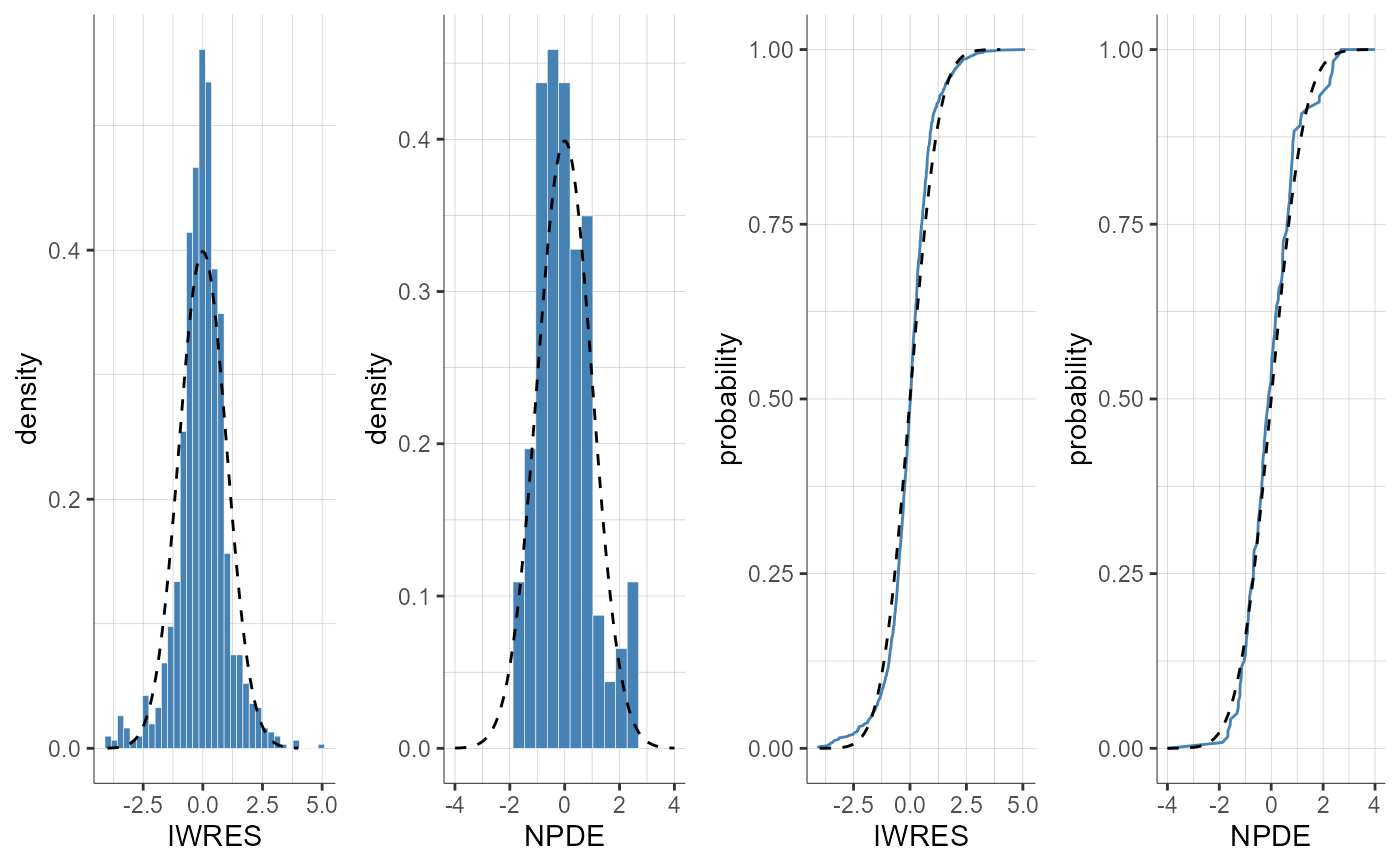
#> TableGrob (1 x 1) "arrange": 1 grobs
#> z cells name grob
#> 1 1 (1-1,1-1) arrange gtable[arrange]
plotResidualsDistribution(residuals = "indiv", settings = list(indivEstimate = "simulated"))
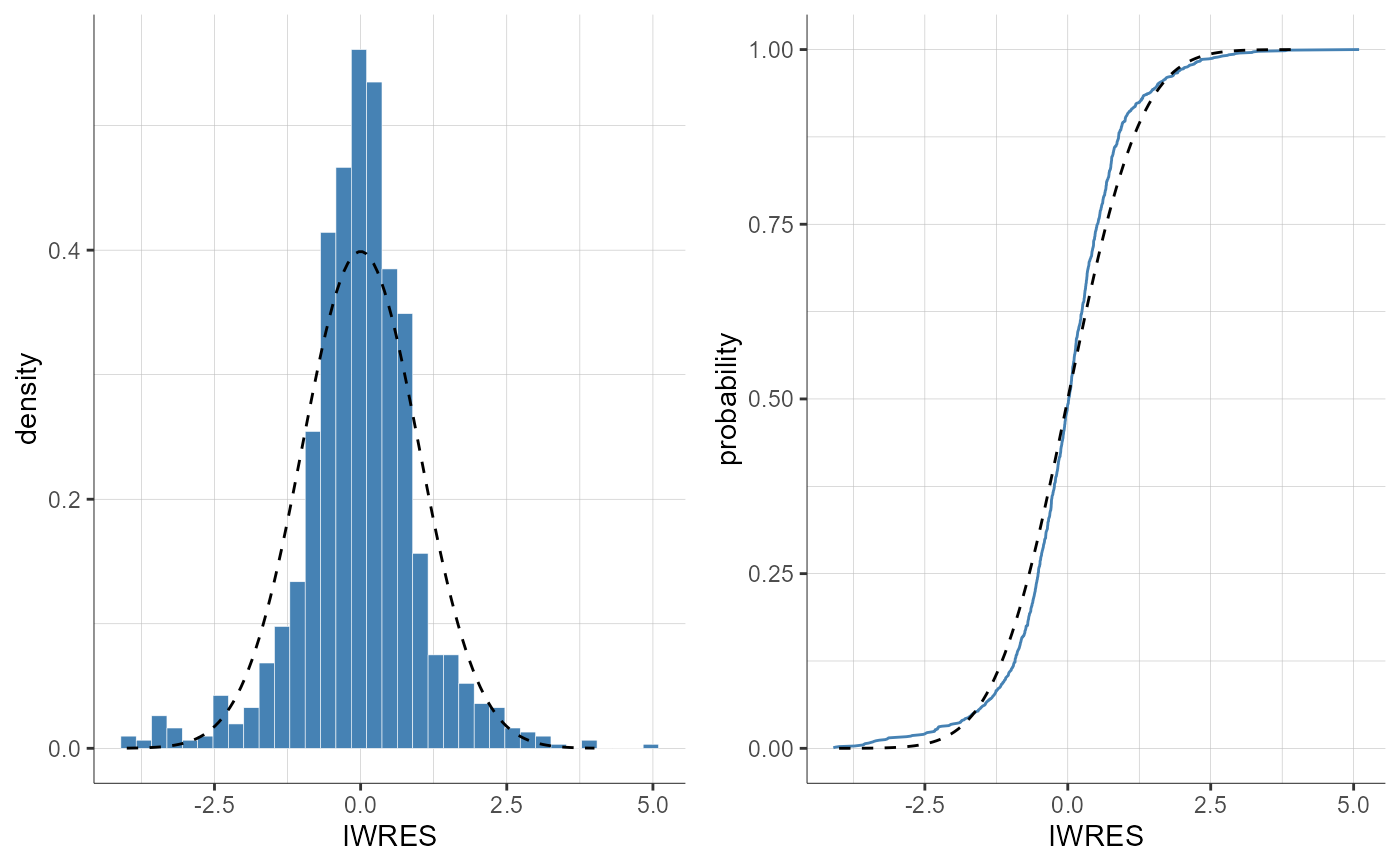
#> TableGrob (1 x 1) "arrange": 1 grobs
#> z cells name grob
#> 1 1 (1-1,1-1) arrange gtable[arrange]
plotResidualsDistribution(residuals = "indiv", settings = list(indivEstimate = "mode"))
#> Warning: Conditional Mode Estimation was not run, individual predictions based on conditional mean computed with MCMC will be displayed instead.
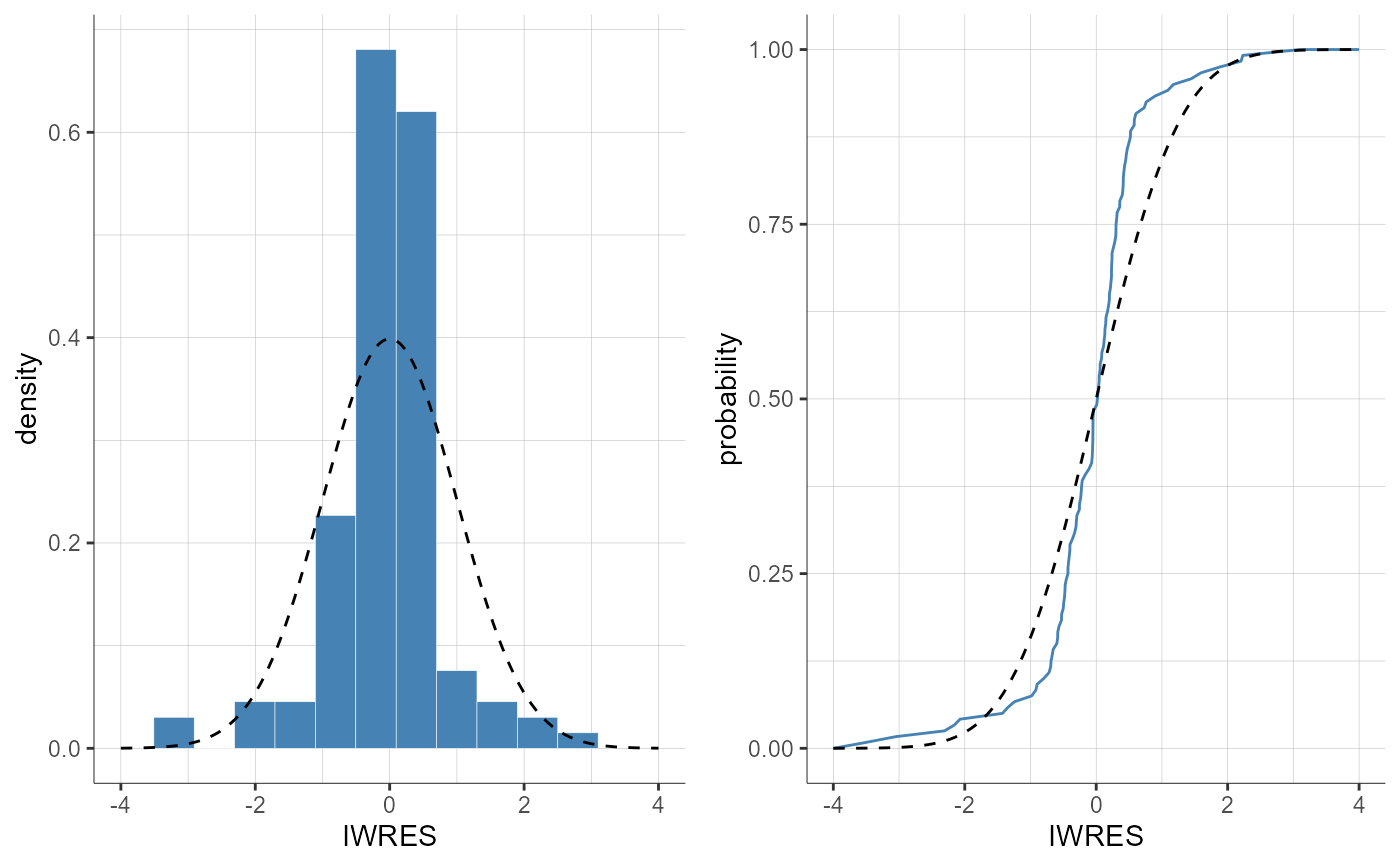
#> TableGrob (1 x 1) "arrange": 1 grobs
#> z cells name grob
#> 1 1 (1-1,1-1) arrange gtable[arrange]
plotResidualsDistribution(residuals = "pop", plots = "pdf")
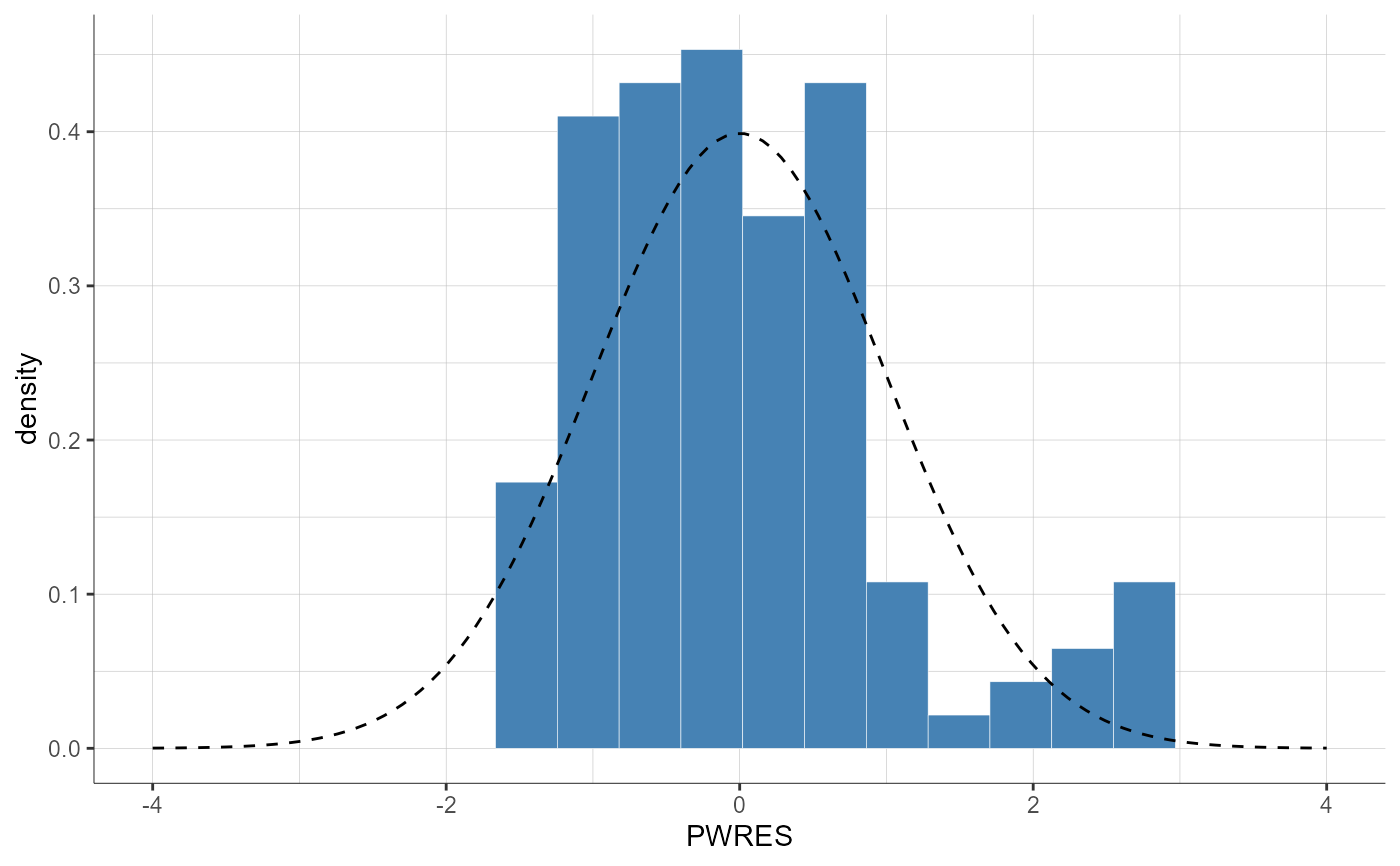
plotResidualsDistribution(residuals = "npde", plots = "cdf")
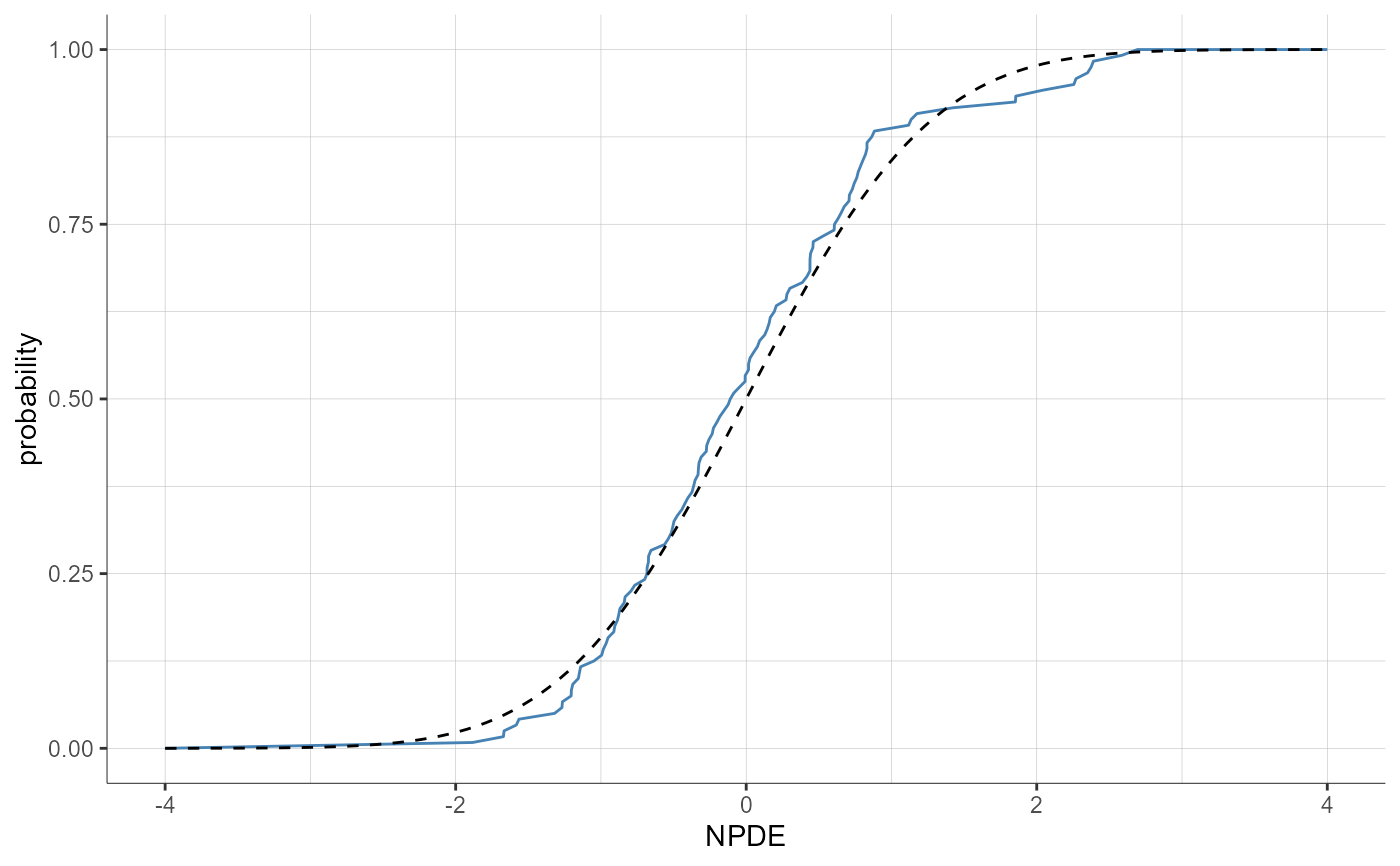
plotResidualsDistribution(stratify = list(filter=list("SEX", "F")))
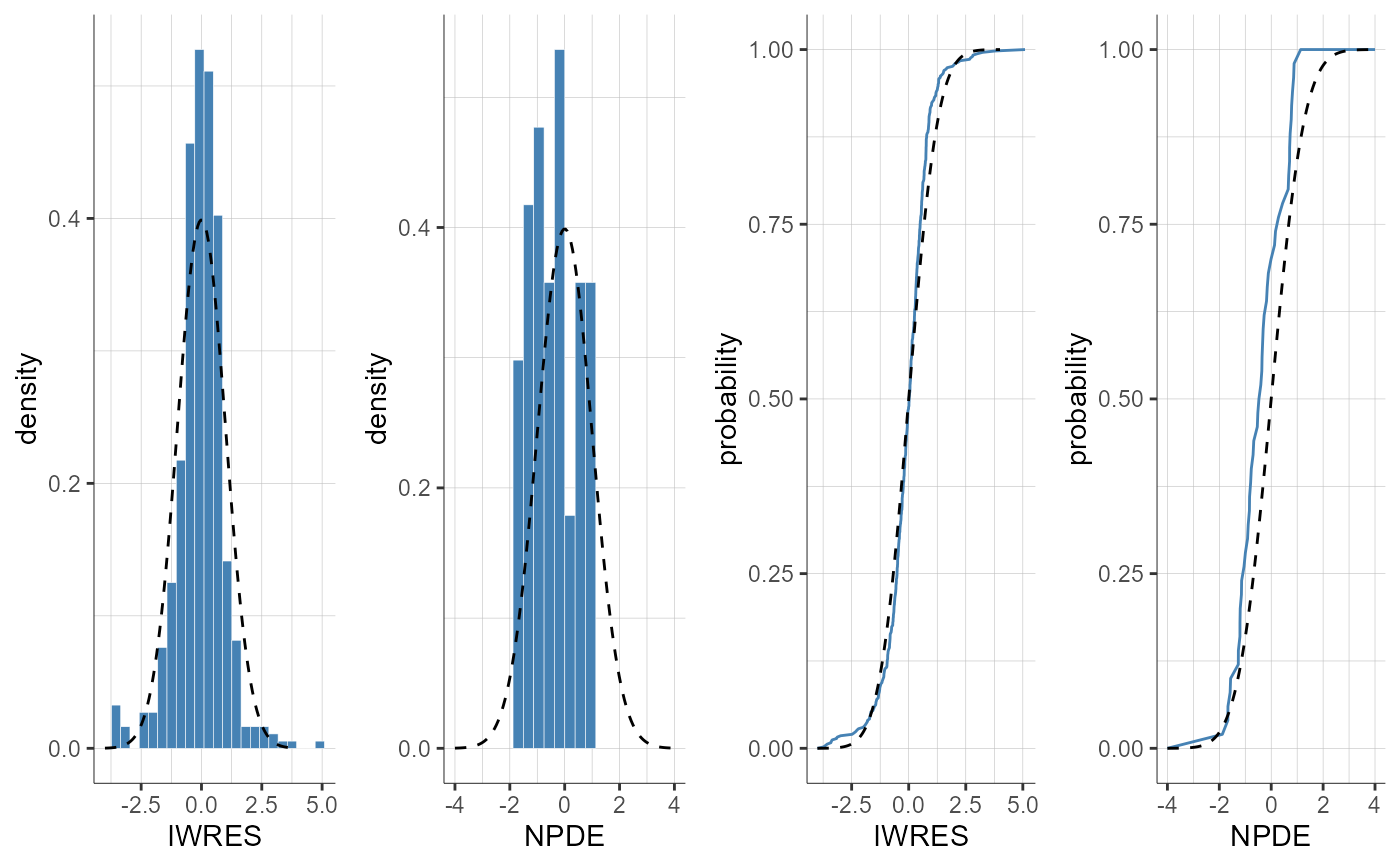
#> TableGrob (1 x 1) "arrange": 1 grobs
#> z cells name grob
#> 1 1 (1-1,1-1) arrange gtable[arrange]
plotResidualsDistribution(stratify = list(split = "WEIGHT",
groups = list(name = "WEIGHT", definition = c(75))))
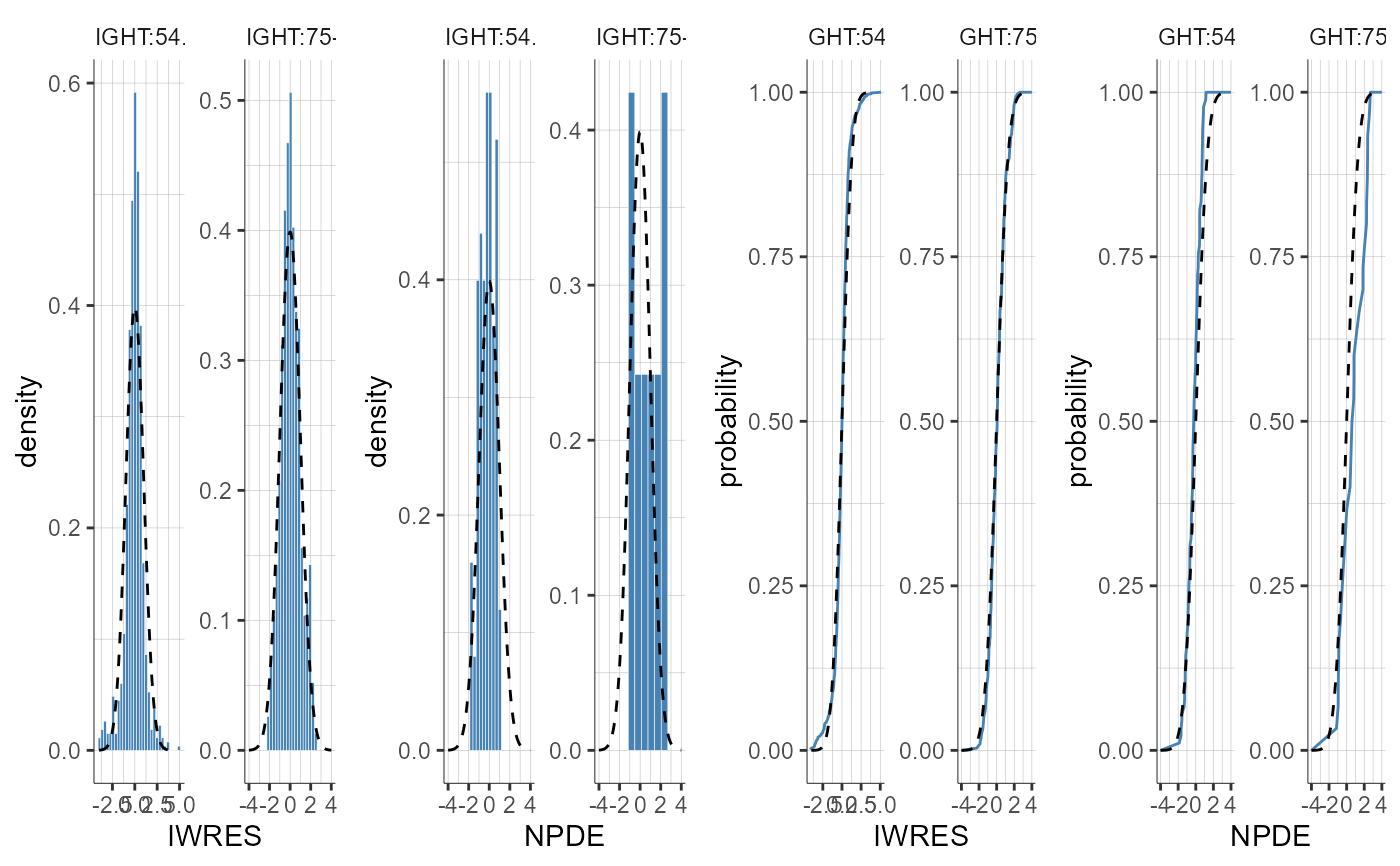
#> TableGrob (1 x 1) "arrange": 1 grobs
#> z cells name grob
#> 1 1 (1-1,1-1) arrange gtable[arrange]
plotResidualsDistribution(residuals = "indiv", settings = list(legend = T),
stratify = list(split = c("SEX", "WEIGHT"),
groups = list(name = "WEIGHT", definition = 70)))
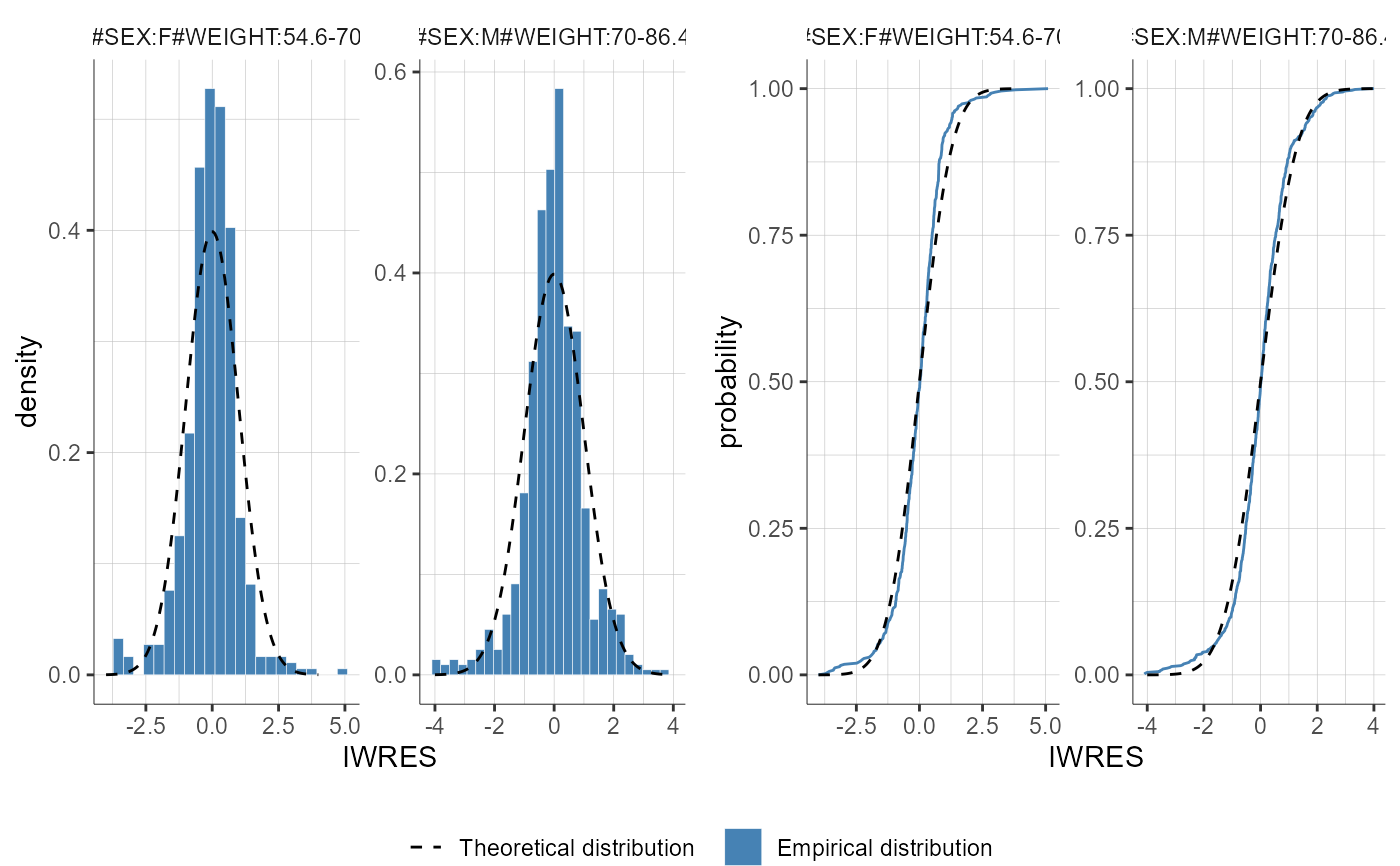
#> TableGrob (2 x 1) "arrange": 2 grobs
#> z cells name grob
#> 1 1 (1-1,1-1) arrange gtable[arrange]
#> 2 2 (2-2,1-1) arrange gtable[guide-box]
plotResidualsDistribution()
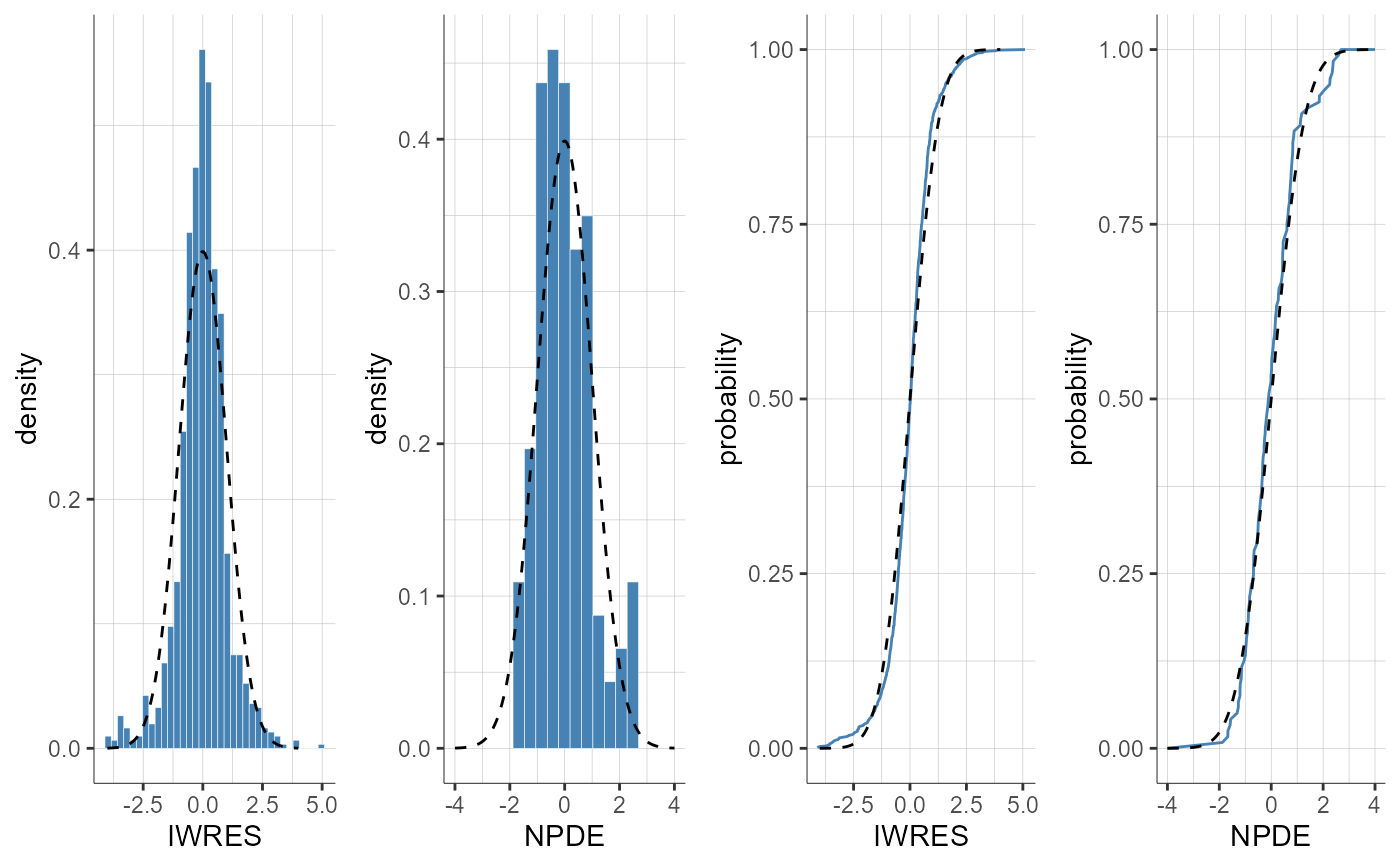
#> TableGrob (1 x 1) "arrange": 1 grobs
#> z cells name grob
#> 1 1 (1-1,1-1) arrange gtable[arrange]
plotResidualsDistribution(residuals = c("indiv", "npde"), settings = list(indivEstimate = "simulated"))
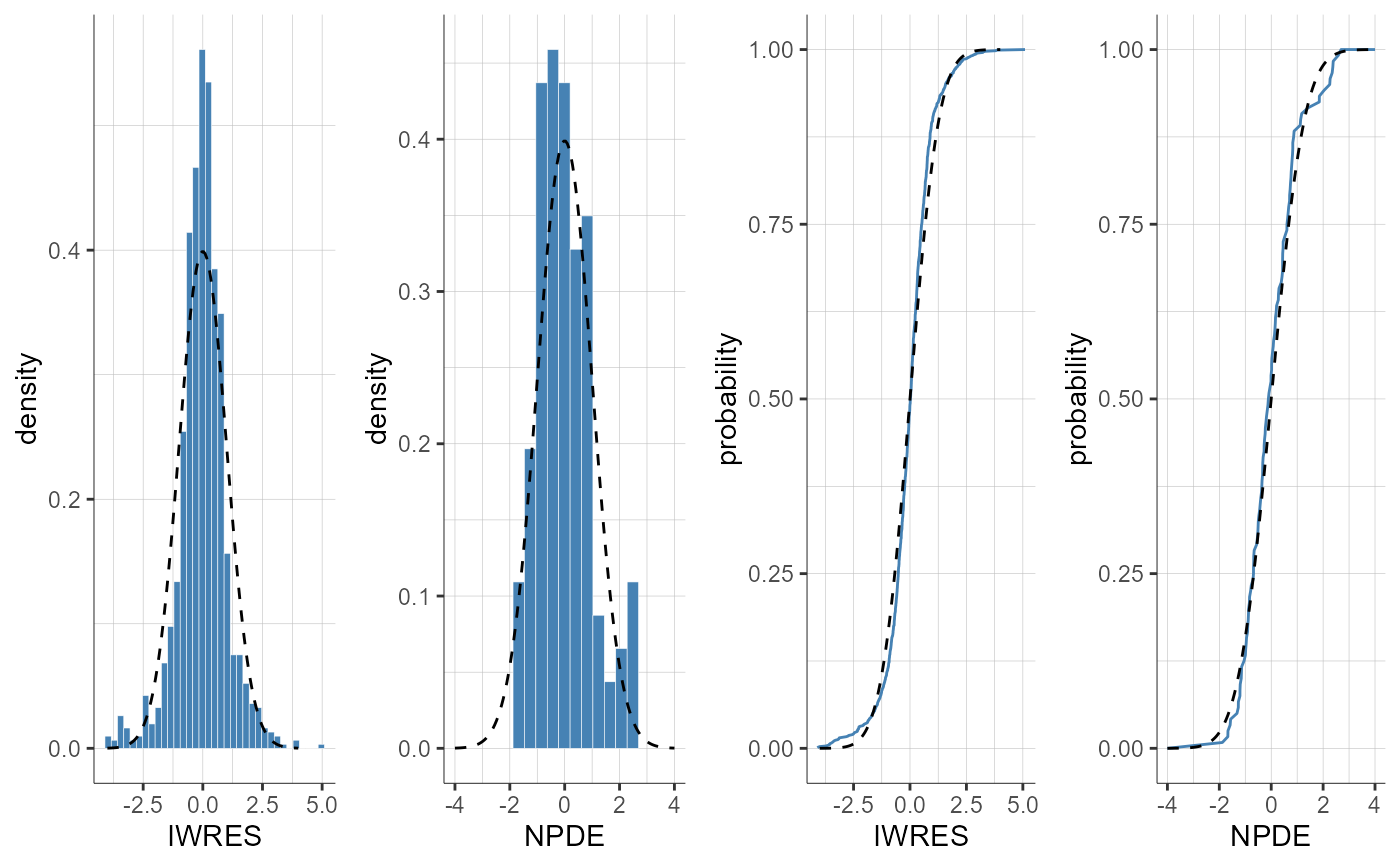
#> TableGrob (1 x 1) "arrange": 1 grobs
#> z cells name grob
#> 1 1 (1-1,1-1) arrange gtable[arrange]
plotResidualsDistribution(residuals = c("pop", "indiv"), settings = list(indivEstimate = "mode"))
#> Warning: Conditional Mode Estimation was not run, individual predictions based on conditional mean computed with MCMC will be displayed instead.
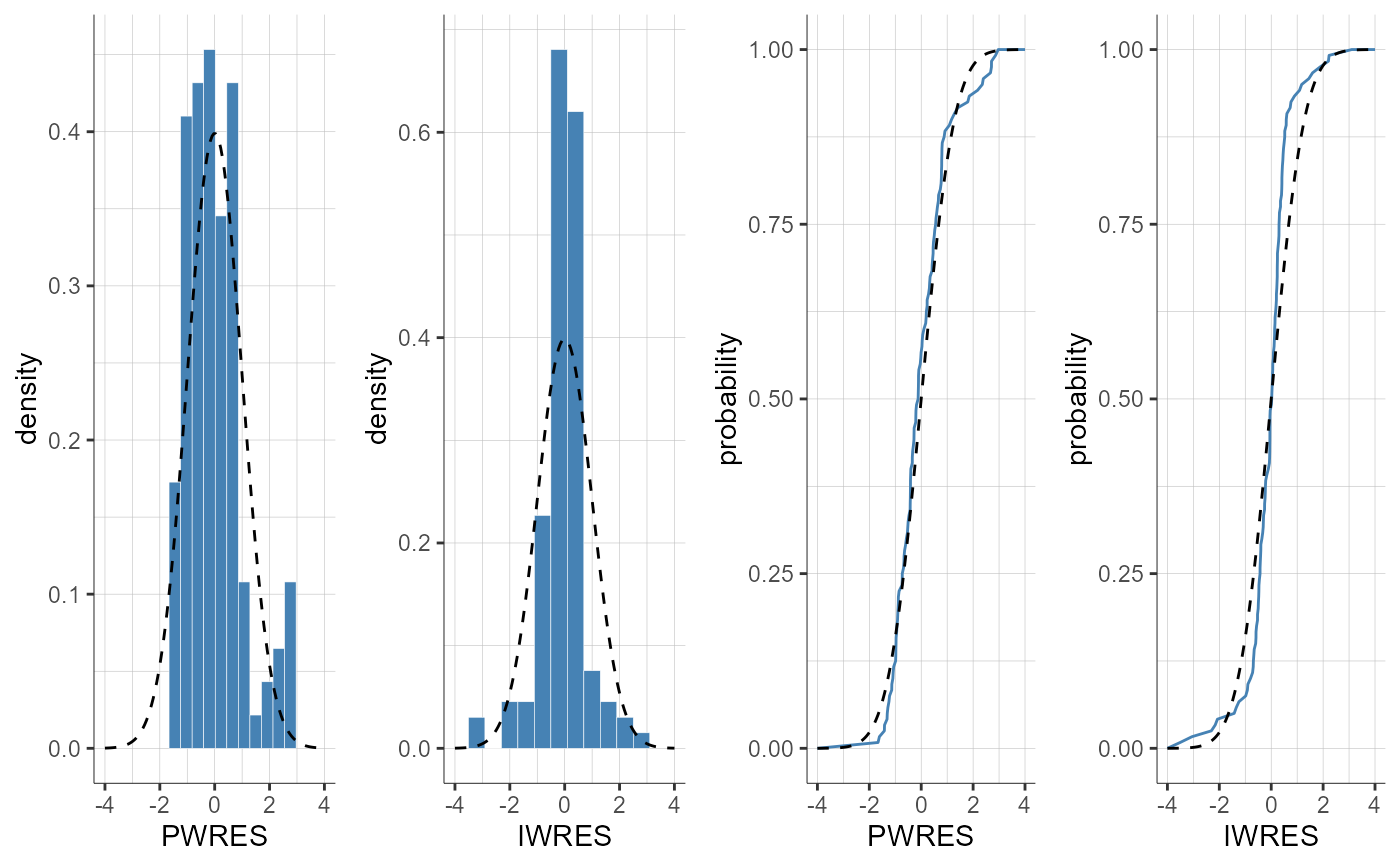
#> TableGrob (1 x 1) "arrange": 1 grobs
#> z cells name grob
#> 1 1 (1-1,1-1) arrange gtable[arrange]
plotResidualsDistribution(plots = c("pdf", "cdf"))
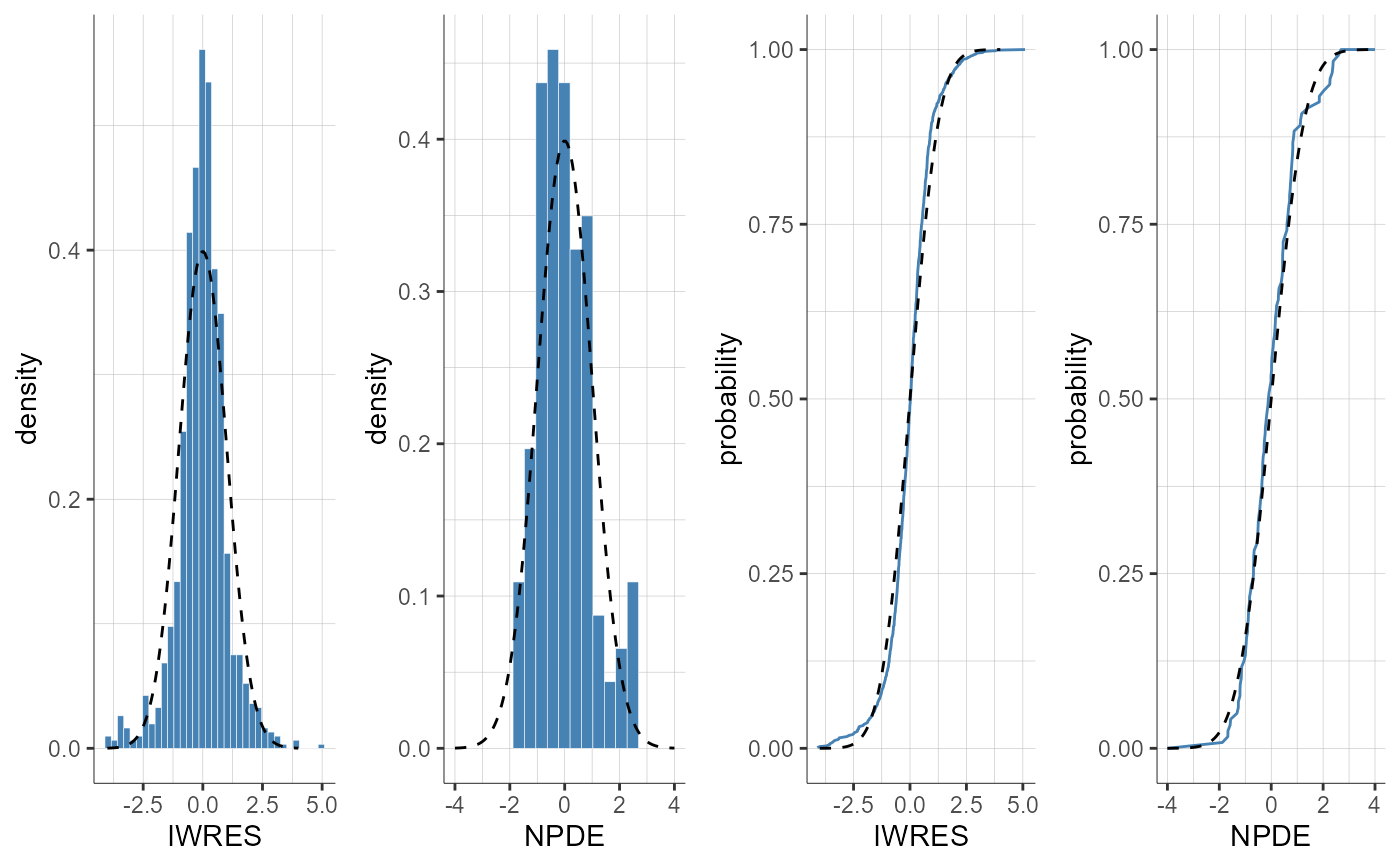
#> TableGrob (1 x 1) "arrange": 1 grobs
#> z cells name grob
#> 1 1 (1-1,1-1) arrange gtable[arrange]
plotResidualsDistribution(plots = c("cdf"))
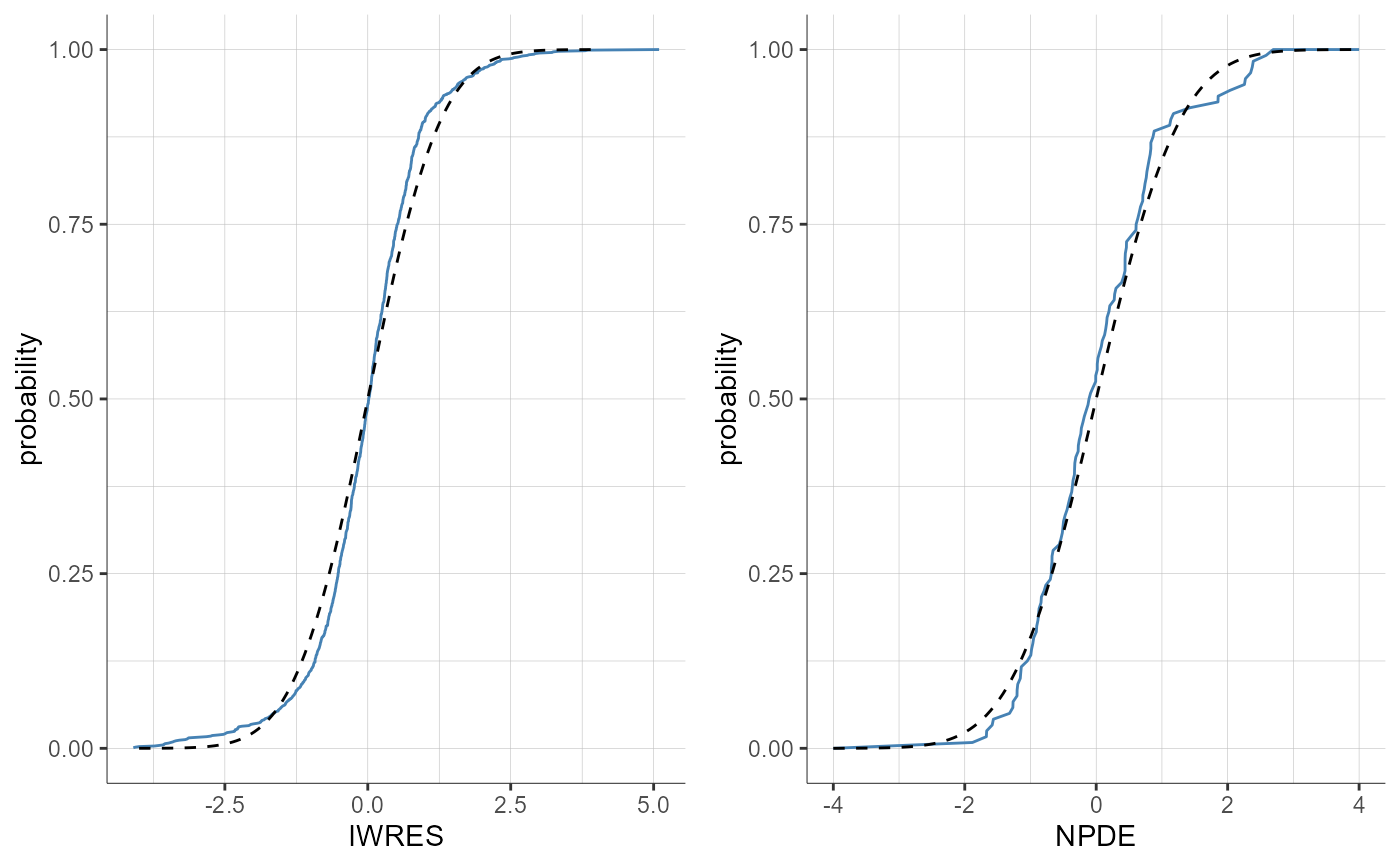
#> TableGrob (1 x 1) "arrange": 1 grobs
#> z cells name grob
#> 1 1 (1-1,1-1) arrange gtable[arrange]
plotResidualsDistribution(residuals = "npde")
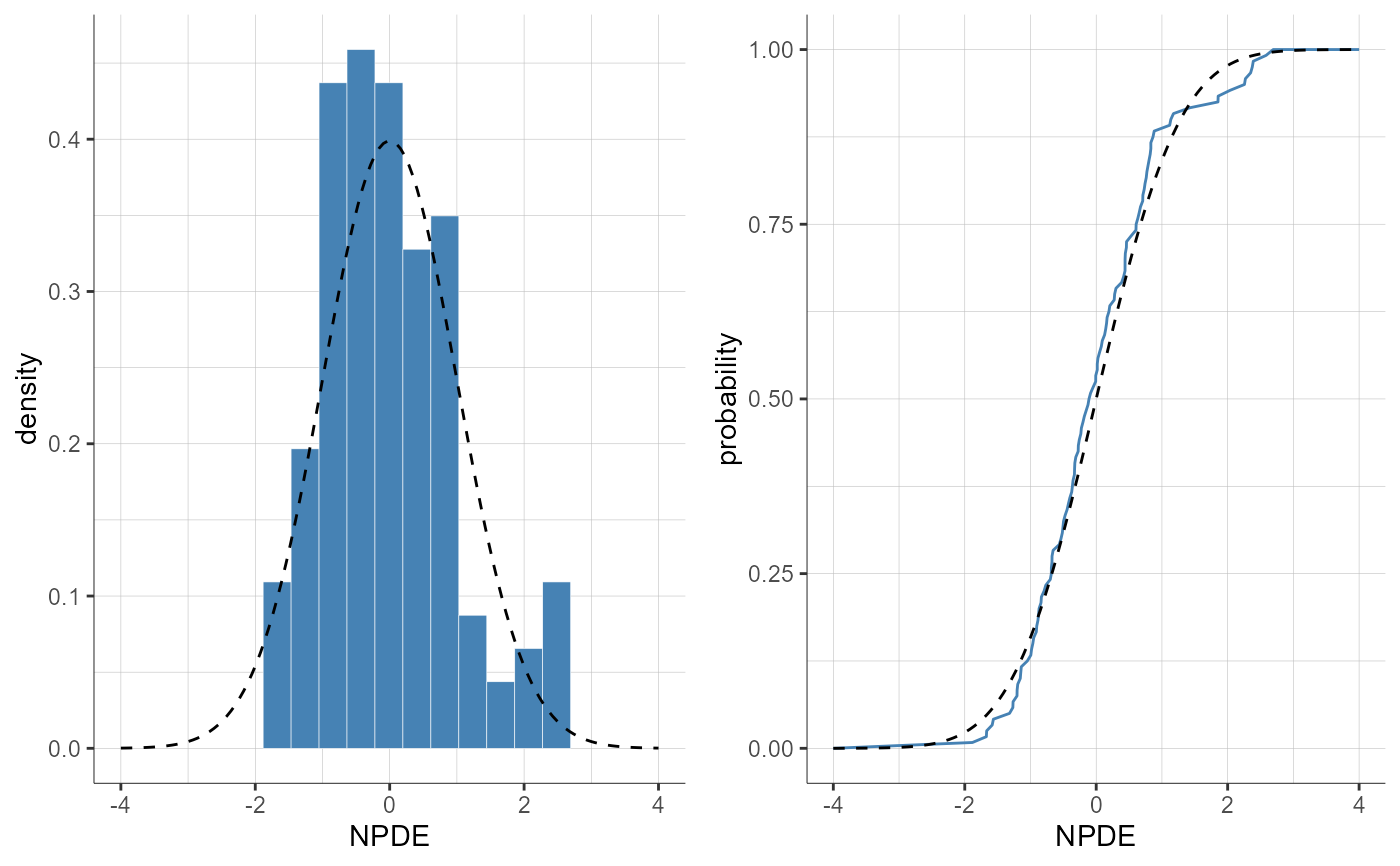
#> TableGrob (1 x 1) "arrange": 1 grobs
#> z cells name grob
#> 1 1 (1-1,1-1) arrange gtable[arrange]